Medical Informatics
Research Article
Using of neural networks to search for errors of patient's positioning on chest X-rays
Alexander Aleksandrovich Borisov1
, Yuri Aleksandrovich Vasiliev2, Anton Vyacheslavovich Vladzimirsky3, Olga Vasil'evna Omelyanskaya4, Serafim Sergeevich Serafim5, Kirill Mikhailovich Arzamasov6
Abstract. The paper presents the results of the application of transfer learning of deep convolutional neural networks for the task of searching for chest X-rays with errors of patient styling and positioning. Evaluated neural network architectures: InceptionV3, Xception, ResNet152V2, InceptionResnetV2, DenseNet201, VGG16, VGG19, MobileNetV2, NASNetLarge. For training and testing we used chest X-rays from open datasets and the unified radiological information service of the city of Moscow. All the models obtained had diagnostic accuracy metrics above 95%, while models based on the ResNet152V2, DenseNet201, VGG16, MobileNetV2 architectures had statistically significantly better metrics than other models. The best absolute values of metrics were shown by the ResNet152V2 model (AUC=0.999, sensitivity=0.987, specificity=0.988, accuracy=0.988, F1 score=0.988). The MobileNetV2 model showed the best processing speed of one study ( ms). The widespread use of the algorithms we have obtained can facilitate the creation of large databases of high-quality medical images, as well as optimize quality control when performing chest X-ray examinations. (In Russian).
Keywords: neural networks, deep learning, quality control, chest X-ray
MSC-2020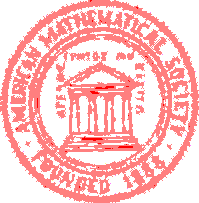
Acknowledgments: The article was prepared within the framework of R&D "Development of a platform for preparing data sets of radiation diagnostic studies" (EGISU No.: 123031500003-8)
For citation: Alexander A. Borisov, Yuri A. Vasiliev, Anton V. Vladzimirsky, Olga V. Omelyanskaya, Serafim S. Serafim, Kirill M. Arzamasov. Using of neural networks to search for errors of patient's positioning on chest X-rays. Program Systems: Theory and Applications, 2023, 14:3, pp. 95–113. (In Russ.). https://psta.psiras.ru/2023/3_95-113.
Full text of article (PDF): https://psta.psiras.ru/read/psta2023_3_95-113.pdf.
The article was submitted 15.04.2023; approved after reviewing 05.05.2023; accepted for publication 18.06.2023; published online 07.10.2023.