Medical Informatics
Research Article
Symptoms extraction and automatic diagnosis prediction from medical clinical records
Yuri Serdyuk1
, Natalia Vlasova2, Seda Momot3
1-3 | Ailamazyan Program Systems Institute of RAS, Ves'kovo, Russia |
1 |
|
Abstract. The paper introduces a system for symptoms extraction from medical clinical records (texts in natural Russian language) and automatic prediction of a diagnosis in the form of the disease title and its ICD-10 code. The system is designed for a restricted domain of 6 pulmonary diseases (chronic obstructive pulmonary disease, pneumonia, bronchial asthma etc) and COVID-19.
Different neural networks are employed for the symptoms extraction by recognizing certain medical entities and relations between them. A classifier based on a neural network is responsible for the automatic diagnosis. An annotated corpus of sentences is created for the training of the neural networks. The principles and rules of the annotation are described. A corpus of texts is used for the training of the classifier.
Both subsystems were tested, the resulting accuracy estimates are provided. The accuracy of diagnosis in the given domain is 88.5%. We also compare our system with similar works on symptom extraction from texts in various languages, as well as on automatic diagnosis, including systems such as ChatGPT. (In Russian).
Keywords: clinical decision support systems, symptom extraction, automatic diagnosis prediction, BERT models, ChatGPT-based systems
MSC-2020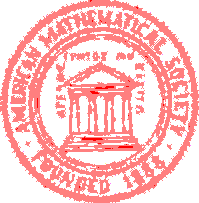
For citation: Yuri Serdyuk, Natalia Vlasova, Seda Momot. Symptoms extraction and automatic diagnosis prediction from medical clinical records. Program Systems: Theory and Applications, 2024, 15:4, pp. 153–181. (In Russ.). https://psta.psiras.ru/2024/4_153-181.
Full text of article (PDF): https://psta.psiras.ru/read/psta2024_4_153-181.pdf.
The article was submitted 03.12.2024; approved after reviewing 27.12.2024; accepted for publication 28.12.2024; published online 28.12.2024.