Artificial intelligence and machine learning
Research Article
Multimodal Stock Price Prediction: A Case Study of the Russian Securities Market
Kasymkhan Usufovich Khubiyev1
, Mikhail Evgenyevich Semenov2
1,2 | Sirius University of Science and Technology, Sirius, Russia |
1 |
|
Abstract. Classical asset price forecasting methods primarily rely on numerical data, such as price time series, trading volumes, limit order book data, and technical analysis indicators. However, the news flow plays a significant role in price formation, making the development of multimodal approaches that combine textual and numerical data for improved prediction accuracy highly relevant.
This paper addresses the problem of forecasting financial asset prices using the multimodal approach that combines candlestick time series and textual news flow data. A unique dataset was collected for the study, which includes time series for 176 Russian stocks traded on the Moscow Exchange and financial news articles in Russian.
For processing textual data, pre-trained models RuBERT and Vikhr-Qwen2.5-0.5b-Instruct (a large language model) were used, while time series and vectorized text data were processed using an LSTM recurrent neural network. The experiments compared models based on a single modality (time series only) and two modalities, as well as various methods for aggregating text vector representations.
Prediction quality was estimated using two key metrics: Accuracy (direction of price movement prediction: up or down) and Mean Absolute Percentage Error (MAPE), which measures the deviation of the predicted price from the true price. The experiments showed that incorporating textual modality reduced the MAPE value by 55%.
The resulting multimodal dataset holds value for the further adaptation of language models in the financial sector. Future research directions include optimizing textual modality parameters, such as the time window, sentiment, and chronological order of news messages. (Linked article texts in English and in Russian).
Keywords: Multimodal Forecasting, Quantitative Fiance, Machine Learning
MSC-2020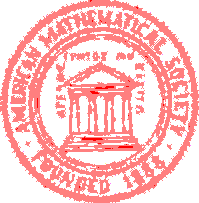
Acknowledgments: This work was supported by the grant of the state program „Scientific and technological development of the `Sirius' Federal Territory“ (Agreement No. 18-03 date 10.09.2024)
For citation: Kasymkhan U. Khubiyev, Mikhail E. Semenov. Multimodal Stock Price Prediction: A Case Study of the Russian Securities Market. Program Systems: Theory and Applications, 2025, 16:1, pp. 83–130. (in Engl. In Russ.). https://psta.psiras.ru/2025/1_83-130.
Full text of bilingual article (PDF): https://psta.psiras.ru/read/psta2025_1_83-130.pdf (Clicking on the flag in the header switches the page language).
The article was submitted 24.12.2024; approved after reviewing 30.01.2025; accepted for publication 27.02.2025; published online 11.03.2025.